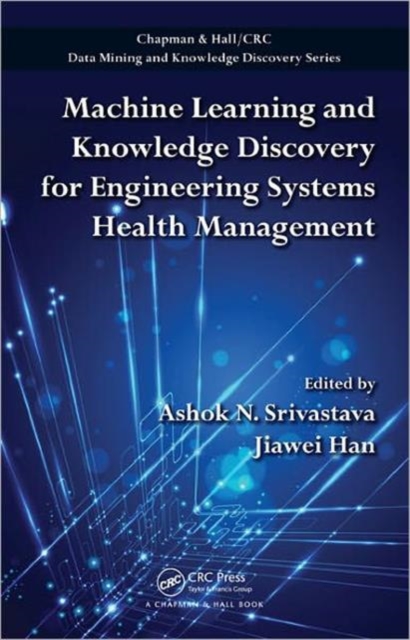
Machine Learning and Knowledge Discovery for Engineering Systems Health Management Hardback
Edited by Ashok N. (Verizon, California, USA) Srivastava, Jiawei (University of Illinois at Urbana-Champaign, USA) Han
Part of the Chapman & Hall/CRC Data Mining and Knowledge Discovery Series series
Hardback
Description
Machine Learning and Knowledge Discovery for Engineering Systems Health Management presents state-of-the-art tools and techniques for automatically detecting, diagnosing, and predicting the effects of adverse events in an engineered system.
With contributions from many top authorities on the subject, this volume is the first to bring together the two areas of machine learning and systems health management. Divided into three parts, the book explains how the fundamental algorithms and methods of both physics-based and data-driven approaches effectively address systems health management.
The first part of the text describes data-driven methods for anomaly detection, diagnosis, and prognosis of massive data streams and associated performance metrics.
It also illustrates the analysis of text reports using novel machine learning approaches that help detect and discriminate between failure modes.
The second part focuses on physics-based methods for diagnostics and prognostics, exploring how these methods adapt to observed data.
It covers physics-based, data-driven, and hybrid approaches to studying damage propagation and prognostics in composite materials and solid rocket motors.
The third part discusses the use of machine learning and physics-based approaches in distributed data centers, aircraft engines, and embedded real-time software systems. Reflecting the interdisciplinary nature of the field, this book shows how various machine learning and knowledge discovery techniques are used in the analysis of complex engineering systems.
It emphasizes the importance of these techniques in managing the intricate interactions within and between the systems to maintain a high degree of reliability.
Information
-
Out of stock
- Format:Hardback
- Pages:502 pages, 32 Tables, black and white; 107 Illustrations, black and white
- Publisher:Taylor & Francis Inc
- Publication Date:16/11/2011
- Category:
- ISBN:9781439841785
Information
-
Out of stock
- Format:Hardback
- Pages:502 pages, 32 Tables, black and white; 107 Illustrations, black and white
- Publisher:Taylor & Francis Inc
- Publication Date:16/11/2011
- Category:
- ISBN:9781439841785