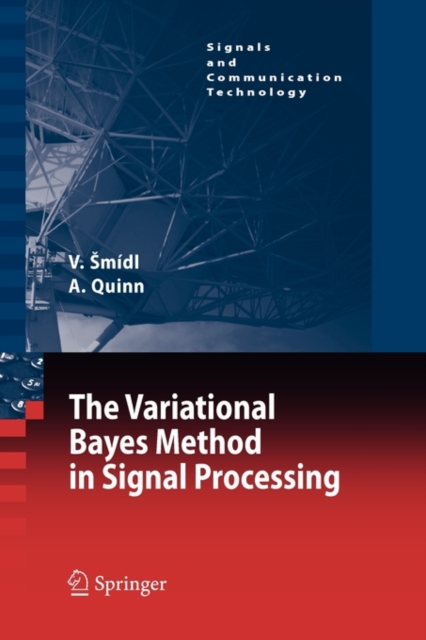
The Variational Bayes Method in Signal Processing Paperback / softback
by Vaclav Smidl, Anthony Quinn
Part of the Signals and Communication Technology series
Paperback / softback
Description
Gaussian linear modelling cannot address current signal processing demands.
In moderncontexts,suchasIndependentComponentAnalysis(ICA),progresshasbeen made speci?cally by imposing non-Gaussian and/or non-linear assumptions.
Hence, standard Wiener and Kalman theories no longer enjoy their traditional hegemony in the ?eld, revealing the standard computational engines for these problems.
In their place, diverse principles have been explored, leading to a consequent diversity in the implied computational algorithms.
The traditional on-line and data-intensive pre- cupations of signal processing continue to demand that these algorithms be tractable.
Increasingly, full probability modelling (the so-called Bayesian approach)-or partial probability modelling using the likelihood function-is the pathway for - sign of these algorithms.
However, the results are often intractable, and so the area of distributional approximation is of increasing relevance in signal processing.
The Expectation-Maximization (EM) algorithm and Laplace approximation, for ex- ple, are standard approaches to handling dif?cult models, but these approximations (certainty equivalence, and Gaussian, respectively) are often too drastic to handle the high-dimensional, multi-modal and/or strongly correlated problems that are - countered. Since the 1990s, stochastic simulation methods have come to dominate Bayesian signal processing.
Markov Chain Monte Carlo (MCMC) sampling, and - lated methods, are appreciated for their ability to simulate possibly high-dimensional distributions to arbitrary levels of accuracy.
More recently, the particle ?ltering - proach has addressed on-line stochastic simulation.
Nevertheless, the wider acce- ability of these methods-and, to some extent, Bayesian signal processing itself- has been undermined by the large computational demands they typically make.
Information
-
Item not Available
- Format:Paperback / softback
- Pages:228 pages, 11 Tables, black and white; 65 Illustrations, black and white; XX, 228 p. 65 illus.
- Publisher:Springer-Verlag Berlin and Heidelberg GmbH & Co. K
- Publication Date:12/02/2010
- Category:
- ISBN:9783642066900
Other Formats
- Hardback from £104.05
- PDF from £76.08
Information
-
Item not Available
- Format:Paperback / softback
- Pages:228 pages, 11 Tables, black and white; 65 Illustrations, black and white; XX, 228 p. 65 illus.
- Publisher:Springer-Verlag Berlin and Heidelberg GmbH & Co. K
- Publication Date:12/02/2010
- Category:
- ISBN:9783642066900