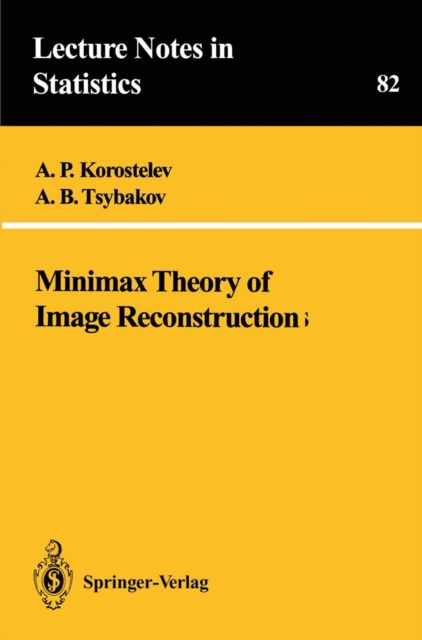
Minimax Theory of Image Reconstruction PDF
by A.P. Korostelev, A.B. Tsybakov
Part of the Lecture Notes in Statistics series
Description
There exists a large variety of image reconstruction methods proposed by different authors (see e. g. Pratt (1978), Rosenfeld and Kak (1982), Marr (1982)).
Selection of an appropriate method for a specific problem in image analysis has been always considered as an art.
How to find the image reconstruction method which is optimal in some sense?
In this book we give an answer to this question using the asymptotic minimax approach in the spirit of Ibragimov and Khasminskii (1980a,b, 1981, 1982), Bretagnolle and Huber (1979), Stone (1980, 1982).
We assume that the image belongs to a certain functional class and we find the image estimators that achieve the best order of accuracy for the worst images in the class.
This concept of optimality is rather rough since only the order of accuracy is optimized.
However, it is useful for comparing various image reconstruction methods.
For example, we show that some popular methods such as simple linewise processing and linear estimation are not optimal for images with sharp edges.
Note that discontinuity of images is an important specific feature appearing in most practical situations where one has to distinguish between the "image domain" and the "background" .
The approach of this book is based on generalization of nonparametric regression and nonparametric change-point techniques.
We discuss these two basic problems in Chapter 1. Chapter 2 is devoted to minimax lower bounds for arbitrary estimators in general statistical models.
Information
-
Download - Immediately Available
- Format:PDF
- Publisher:Springer New York
- Publication Date:06/12/2012
- Category:
- ISBN:9781461227120
Other Formats
- Paperback / softback from £81.69
Information
-
Download - Immediately Available
- Format:PDF
- Publisher:Springer New York
- Publication Date:06/12/2012
- Category:
- ISBN:9781461227120