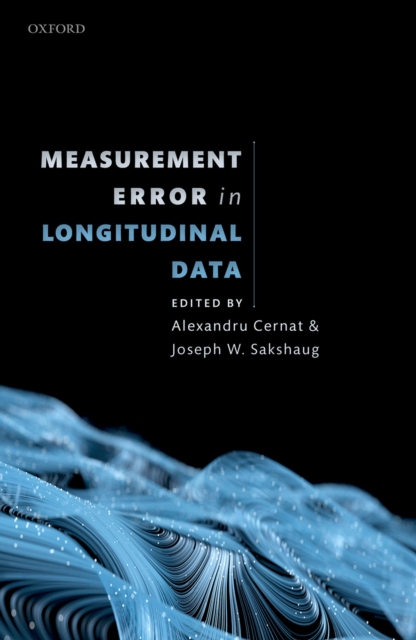
Measurement Error in Longitudinal Data PDF
Edited by Alexandru Cernat, Joseph W. Sakshaug
Description
Longitudinal data is essential for understanding how the world around us changes.
Most theories in the social sciences and elsewhere have a focus on change, be it of individuals, of countries, of organizations, or of systems, and this is reflected in the myriad of longitudinal data that are being collected using large panel surveys.
This type of data collection has been made easier in the age of Big Data and with the rise of social media.
Yet our measurements of theworld are often imperfect, and longitudinal data is vulnerable to measurement errors which can lead to flawed and misleading conclusions. Measurement Error in Longitudinal Data tackles the important issue of how to investigate change in the context of imperfect data.
It compiles the latest advances in estimating change in the presence of measurement error from several fields and covers the entire process, from the best ways of collecting longitudinal data, to statistical models to estimate change under uncertainty, to examples of researchers applying these methods in the real world. This book introduces the essential issues of longitudinal data collection, such as memory effects, panel conditioning (or mere measurement effects), the use of administrative data, and the collection of multi-mode longitudinal data.
It also presents some of the most important models used in this area, including quasi-simplex models, latent growth models, latent Markov chains, and equivalence/DIF testing.
Finally, the use of vignettes in the context of longitudinal data and estimation methodsfor multilevel models of change in the presence of measurement error are also discussed.
Information
-
Download - Immediately Available
- Format:PDF
- Pages:304 pages
- Publisher:OUP Oxford
- Publication Date:18/03/2021
- Category:
- ISBN:9780192603982
Information
-
Download - Immediately Available
- Format:PDF
- Pages:304 pages
- Publisher:OUP Oxford
- Publication Date:18/03/2021
- Category:
- ISBN:9780192603982