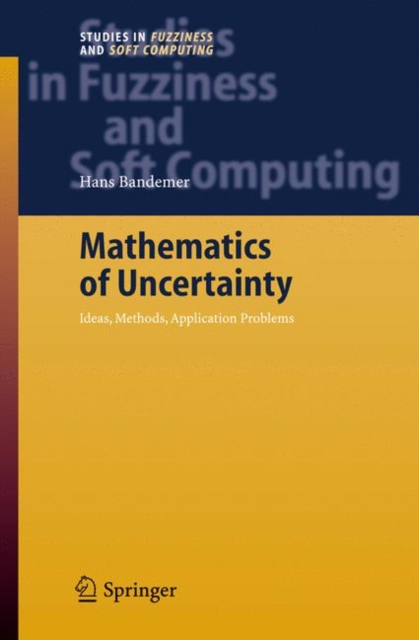
Rule-Based Evolutionary Online Learning Systems : A Principled Approach to LCS Analysis and Design PDF
by Martin V. Butz
Part of the Studies in Fuzziness and Soft Computing series
Description
Rule-basedevolutionaryonlinelearningsystems,oftenreferredtoasMichig- style learning classi?er systems (LCSs), were proposed nearly thirty years ago (Holland, 1976; Holland, 1977) originally calling them cognitive systems.
LCSs combine the strength of reinforcement learning with the generali- tion capabilities of genetic algorithms promising a ?exible, online general- ing, solely reinforcement dependent learning system.
However, despite several initial successful applications of LCSs and their interesting relations with a- mal learning and cognition, understanding of the systems remained somewhat obscured.
Questions concerning learning complexity or convergence remained unanswered.
Performance in di?erent problem types, problem structures, c- ceptspaces,andhypothesisspacesstayednearlyunpredictable.
Thisbookhas the following three major objectives: (1) to establish a facetwise theory - proachforLCSsthatpromotessystemanalysis,understanding,anddesign;(2) to analyze, evaluate, and enhance the XCS classi?er system (Wilson, 1995) by the means of the facetwise approach establishing a fundamental XCS learning theory; (3) to identify both the major advantages of an LCS-based learning approach as well as the most promising potential application areas.
Achieving these three objectives leads to a rigorous understanding of LCS functioning that enables the successful application of LCSs to diverse problem types and problem domains.
The quantitative analysis of XCS shows that the inter- tive, evolutionary-based online learning mechanism works machine learning competitively yielding a low-order polynomial learning complexity.
Moreover, the facetwise analysis approach facilitates the successful design of more - vanced LCSs including Holland's originally envisioned cognitivesystems.
Martin V.
Information
-
Download - Immediately Available
- Format:PDF
- Publisher:Springer Berlin Heidelberg
- Publication Date:04/01/2006
- Category:
- ISBN:9783540312314
Other Formats
- Hardback from £89.99
Information
-
Download - Immediately Available
- Format:PDF
- Publisher:Springer Berlin Heidelberg
- Publication Date:04/01/2006
- Category:
- ISBN:9783540312314