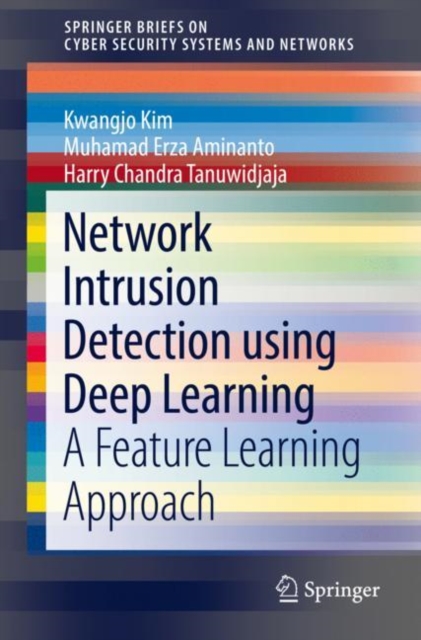
Network Intrusion Detection using Deep Learning : A Feature Learning Approach Paperback / softback
by Kwangjo Kim, Muhamad Erza Aminanto, Harry Chandra Tanuwidjaja
Part of the SpringerBriefs on Cyber Security Systems and Networks series
Paperback / softback
Description
This book presents recent advances in intrusion detection systems (IDSs) using state-of-the-art deep learning methods.
It also provides a systematic overview of classical machine learning and the latest developments in deep learning. In particular, it discusses deep learning applications in IDSs in different classes: generative, discriminative, and adversarial networks.
Moreover, it compares various deep learning-based IDSs based on benchmarking datasets.
The book also proposes two novel feature learning models: deep feature extraction and selection (D-FES) and fully unsupervised IDS.
Further challenges and research directions are presented at the end of the book.
Offering a comprehensive overview of deep learning-based IDS, the book is a valuable reerence resource for undergraduate and graduate students, as well as researchers and practitioners interested in deep learning and intrusion detection.
Further, the comparison of various deep-learning applications helps readers gain a basic understanding of machine learning, and inspires applications in IDS and other related areas in cybersecurity.
Information
-
Out of stock
- Format:Paperback / softback
- Pages:79 pages, 11 Illustrations, color; 19 Illustrations, black and white; XVII, 79 p. 30 illus., 11 illu
- Publisher:Springer Verlag, Singapore
- Publication Date:02/10/2018
- Category:
- ISBN:9789811314438
Information
-
Out of stock
- Format:Paperback / softback
- Pages:79 pages, 11 Illustrations, color; 19 Illustrations, black and white; XVII, 79 p. 30 illus., 11 illu
- Publisher:Springer Verlag, Singapore
- Publication Date:02/10/2018
- Category:
- ISBN:9789811314438