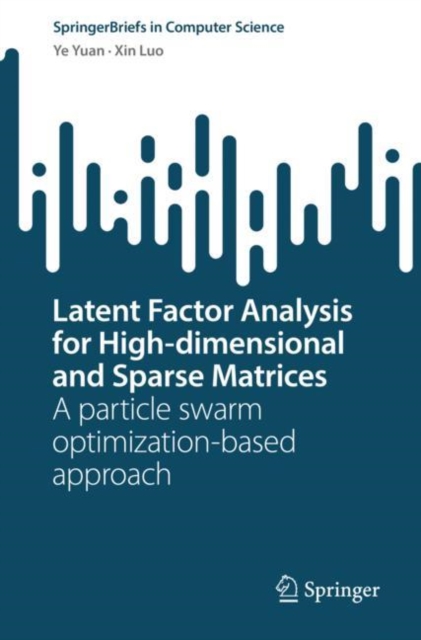
Latent Factor Analysis for High-dimensional and Sparse Matrices : A particle swarm optimization-based approach Paperback / softback
by Ye Yuan, Xin Luo
Part of the SpringerBriefs in Computer Science series
Paperback / softback
Description
Latent factor analysis models are an effective type of machine learning model for addressing high-dimensional and sparse matrices, which are encountered in many big-data-related industrial applications.
The performance of a latent factor analysis model relies heavily on appropriate hyper-parameters.
However, most hyper-parameters are data-dependent, and using grid-search to tune these hyper-parameters is truly laborious and expensive in computational terms.
Hence, how to achieve efficient hyper-parameter adaptation for latent factor analysis models has become a significant question.This is the first book to focus on how particle swarm optimization can be incorporated into latent factor analysis for efficient hyper-parameter adaptation, an approach that offers high scalability in real-world industrial applications. The book will help students, researchers and engineers fully understand the basic methodologies of hyper-parameter adaptation via particle swarm optimization in latent factor analysis models.
Further, it will enable them to conduct extensive research and experiments on the real-world applications of the content discussed.
Information
-
Available to Order - This title is available to order, with delivery expected within 2 weeks
- Format:Paperback / softback
- Pages:92 pages, 1 Illustrations, black and white; VIII, 92 p. 1 illus.
- Publisher:Springer Verlag, Singapore
- Publication Date:16/11/2022
- Category:
- ISBN:9789811967023
Information
-
Available to Order - This title is available to order, with delivery expected within 2 weeks
- Format:Paperback / softback
- Pages:92 pages, 1 Illustrations, black and white; VIII, 92 p. 1 illus.
- Publisher:Springer Verlag, Singapore
- Publication Date:16/11/2022
- Category:
- ISBN:9789811967023