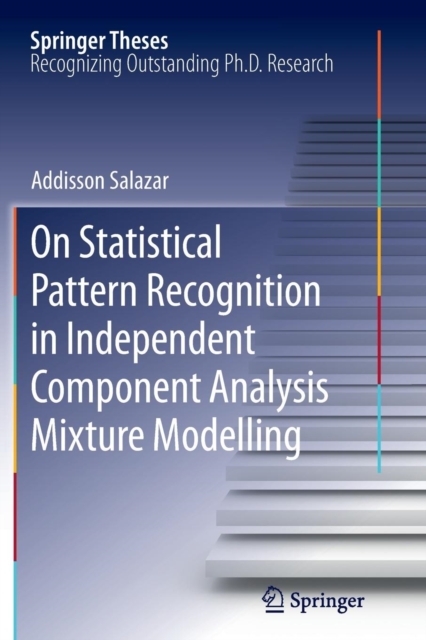
On Statistical Pattern Recognition in Independent Component Analysis Mixture Modelling Paperback / softback
by ADDISSON SALAZAR
Part of the Springer Theses series
Paperback / softback
Description
A natural evolution of statistical signal processing, in connection with the progressive increase in computational power, has been exploiting higher-order information.
Thus, high-order spectral analysis and nonlinear adaptive filtering have received the attention of many researchers.
One of the most successful techniques for non-linear processing of data with complex non-Gaussian distributions is the independent component analysis mixture modelling (ICAMM).
This thesis defines a novel formalism for pattern recognition and classification based on ICAMM, which unifies a certain number of pattern recognition tasks allowing generalization.
The versatile and powerful framework developed in this work can deal with data obtained from quite different areas, such as image processing, impact-echo testing, cultural heritage, hypnograms analysis, web-mining and might therefore be employed to solve many different real-world problems.
Information
-
Item not Available
- Format:Paperback / softback
- Pages:186 pages, XXII, 186 p.
- Publisher:Springer-Verlag Berlin and Heidelberg GmbH & Co. K
- Publication Date:09/08/2014
- Category:
- ISBN:9783642428753
Other Formats
- Hardback from £95.55
- PDF from £76.08
Information
-
Item not Available
- Format:Paperback / softback
- Pages:186 pages, XXII, 186 p.
- Publisher:Springer-Verlag Berlin and Heidelberg GmbH & Co. K
- Publication Date:09/08/2014
- Category:
- ISBN:9783642428753