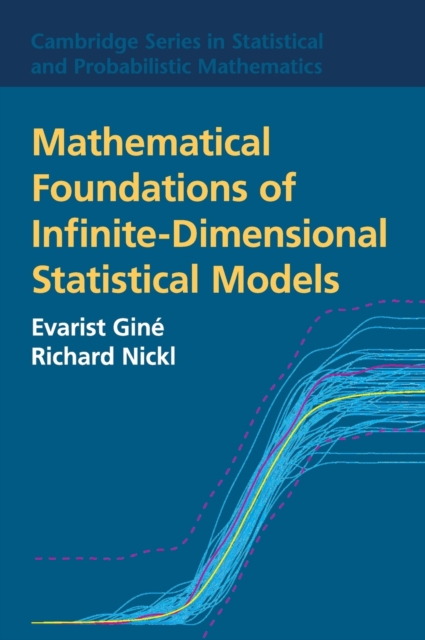
Mathematical Foundations of Infinite-Dimensional Statistical Models Hardback
by Evarist (University of Connecticut) Gine, Richard (University of Cambridge) Nickl
Part of the Cambridge Series in Statistical and Probabilistic Mathematics series
Hardback
Description
In nonparametric and high-dimensional statistical models, the classical Gauss-Fisher-Le Cam theory of the optimality of maximum likelihood estimators and Bayesian posterior inference does not apply, and new foundations and ideas have been developed in the past several decades.
This book gives a coherent account of the statistical theory in infinite-dimensional parameter spaces.
The mathematical foundations include self-contained 'mini-courses' on the theory of Gaussian and empirical processes, on approximation and wavelet theory, and on the basic theory of function spaces.
The theory of statistical inference in such models - hypothesis testing, estimation and confidence sets - is then presented within the minimax paradigm of decision theory.
This includes the basic theory of convolution kernel and projection estimation, but also Bayesian nonparametrics and nonparametric maximum likelihood estimation.
In the final chapter, the theory of adaptive inference in nonparametric models is developed, including Lepski's method, wavelet thresholding, and adaptive inference for self-similar functions.
Information
-
Out of stock
- Format:Hardback
- Pages:720 pages
- Publisher:Cambridge University Press
- Publication Date:18/11/2015
- Category:
- ISBN:9781107043169
Information
-
Out of stock
- Format:Hardback
- Pages:720 pages
- Publisher:Cambridge University Press
- Publication Date:18/11/2015
- Category:
- ISBN:9781107043169