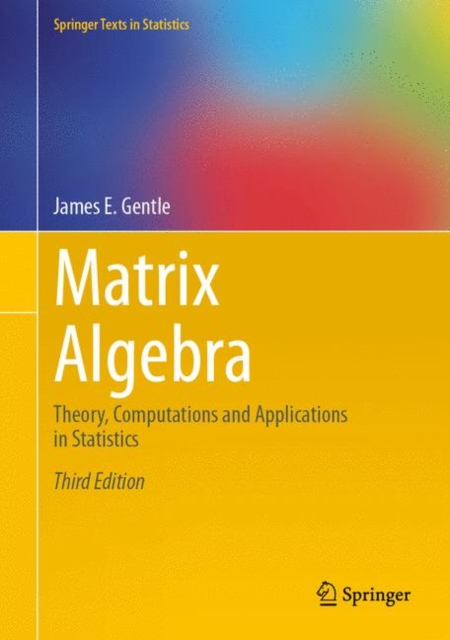
Matrix Algebra : Theory, Computations and Applications in Statistics Hardback
by James E. Gentle
Part of the Springer Texts in Statistics series
Hardback
Description
This book presents the theory of matrix algebra for statistical applications, explores various types of matrices encountered in statistics, and covers numerical linear algebra.
Matrix algebra is one of the most important areas of mathematics in data science and in statistical theory, and previous editions had essential updates and comprehensive coverage on critical topics in mathematics. This 3rd edition offers a self-contained description of relevant aspects of matrix algebra for applications in statistics.
It begins with fundamental concepts of vectors and vector spaces; covers basic algebraic properties of matrices and analytic properties of vectors and matrices in multivariate calculus; and concludes with a discussion on operations on matrices, in solutions of linear systems and in eigenanalysis.
It also includes discussions of the R software package, with numerous examples and exercises. Matrix Algebra considers various types of matrices encountered in statistics, such as projection matrices and positive definite matrices, and describes special properties of those matrices; as well as describing various applications of matrix theory in statistics, including linear models, multivariate analysis, and stochastic processes.
It begins with a discussion of the basics of numerical computations and goes on to describe accurate and efficient algorithms for factoring matrices, how to solve linear systems of equations, and the extraction of eigenvalues and eigenvectors.
It covers numerical linear algebra—one of the most important subjects in the field of statistical computing.
The content includes greater emphases on R, and extensive coverage of statistical linear models. Matrix Algebra is ideal for graduate and advanced undergraduate students, or as a supplementary text for courses in linear models or multivariate statistics.
It’s also ideal for use in a course in statistical computing, or as a supplementary text forvarious courses that emphasize computations.
Information
-
Only a few left - usually despatched within 24 hours
- Format:Hardback
- Pages:693 pages, 107 Illustrations, black and white; XXXII, 693 p. 107 illus.
- Publisher:Springer International Publishing AG
- Publication Date:07/03/2024
- Category:
- ISBN:9783031421433
Other Formats
- Paperback / softback from £56.35
- PDF from £76.08
£89.99
£80.59
Information
-
Only a few left - usually despatched within 24 hours
- Format:Hardback
- Pages:693 pages, 107 Illustrations, black and white; XXXII, 693 p. 107 illus.
- Publisher:Springer International Publishing AG
- Publication Date:07/03/2024
- Category:
- ISBN:9783031421433