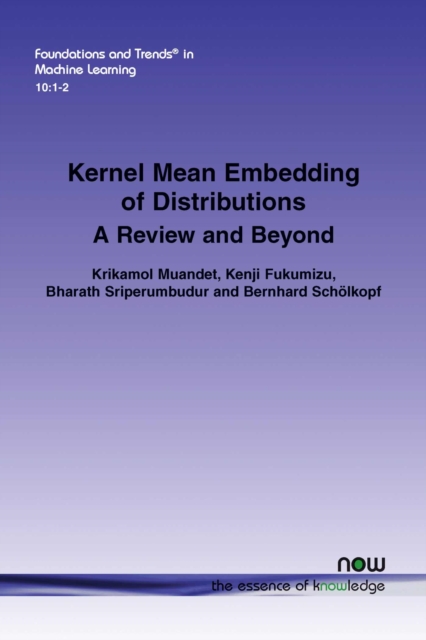
Kernel Mean Embedding of Distributions : A Review and Beyond Paperback / softback
by KRIKAMOL MUANDET, Kenji Fukumizu, Bharath Sriperumbudur, Bernhard Scholkopf
Part of the Foundations and Trends in Machine Learning series
Paperback / softback
Description
A Hilbert space embedding of a distribution-in short, a kernel mean embedding-has recently emerged as a powerful tool for machine learning and statistical inference.
The basic idea behind this framework is to map distributions into a reproducing kernel Hilbert space (RKHS) in which the whole arsenal of kernel methods can be extended to probability measures.
It can be viewed as a generalization of the original "feature map" common to support vector machines (SVMs) and other kernel methods.
In addition to the classical applications of kernel methods, the kernel mean embedding has found novel applications in fields ranging from probabilistic modelling to statistical inference, causal discovery, and deep learning.
Kernel Mean Embedding of Distributions: A Review and Beyond provides a comprehensive review of existing work and recent advances in this research area, and to discuss some of the most challenging issues and open problems that could potentially lead to new research directions.
The targeted audience includes graduate students and researchers in machine learning and statistics who are interested in the theory and applications of kernel mean embeddings.
Information
-
Out of stock
- Format:Paperback / softback
- Pages:154 pages
- Publisher:now publishers Inc
- Publication Date:28/06/2017
- Category:
- ISBN:9781680832884
Information
-
Out of stock
- Format:Paperback / softback
- Pages:154 pages
- Publisher:now publishers Inc
- Publication Date:28/06/2017
- Category:
- ISBN:9781680832884