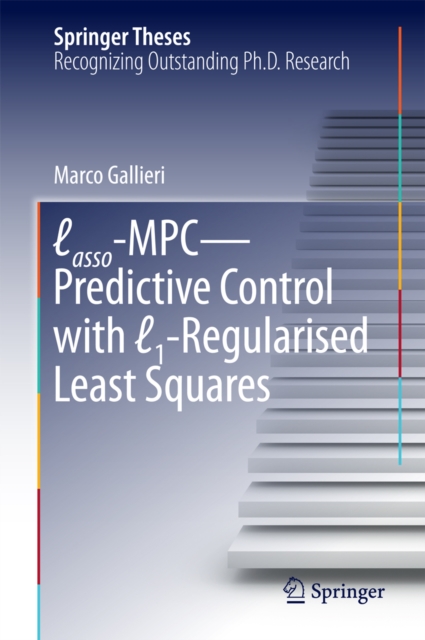
Lasso-MPC - Predictive Control with l1-Regularised Least Squares PDF
by Marco Gallieri
Part of the Springer Theses series
Description
This thesis proposes a novel Model Predictive Control (MPC) strategy, which modifies the usual MPC cost function in order to achieve a desirable sparse actuation.
It features an l1-regularised least squares loss function, in which the control error variance competes with the sum of input channels magnitude (or slew rate) over the whole horizon length.
While standard control techniques lead to continuous movements of all actuators, this approach enables a selected subset of actuators to be used, the others being brought into play in exceptional circumstances.
The same approach can also be used to obtain asynchronous actuator interventions, so that control actions are only taken in response to large disturbances.
This thesis presents a straightforward and systematic approach to achieving these practical properties, which are ignored by mainstream control theory.
Information
-
Download - Immediately Available
- Format:PDF
- Publisher:Springer International Publishing
- Publication Date:31/03/2016
- Category:
- ISBN:9783319279633
Other Formats
- Paperback / softback from £89.99
Information
-
Download - Immediately Available
- Format:PDF
- Publisher:Springer International Publishing
- Publication Date:31/03/2016
- Category:
- ISBN:9783319279633