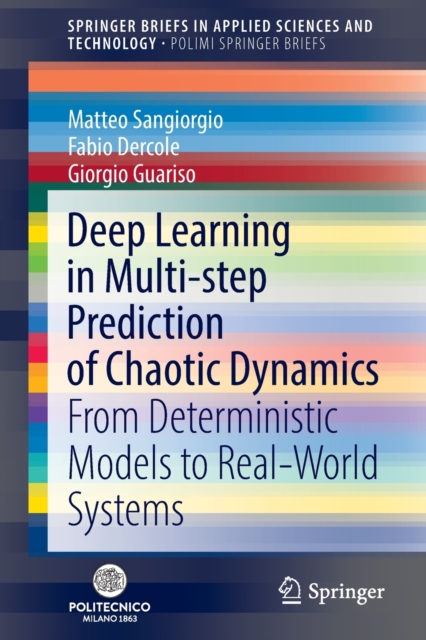
Deep Learning in Multi-step Prediction of Chaotic Dynamics : From Deterministic Models to Real-World Systems Paperback / softback
by Matteo Sangiorgio, Fabio Dercole, Giorgio Guariso
Part of the SpringerBriefs in Applied Sciences and Technology series
Paperback / softback
Description
The book represents the first attempt to systematically deal with the use of deep neural networks to forecast chaotic time series.
Differently from most of the current literature, it implements a multi-step approach, i.e., the forecast of an entire interval of future values.
This is relevant for many applications, such as model predictive control, that requires predicting the values for the whole receding horizon.
Going progressively from deterministic models with different degrees of complexity and chaoticity to noisy systems and then to real-world cases, the book compares the performances of various neural network architectures (feed-forward and recurrent).
It also introduces an innovative and powerful approach for training recurrent structures specific for sequence-to-sequence tasks.
The book also presents one of the first attempts in the context of environmental time series forecasting of applying transfer-learning techniques such as domain adaptation.
Information
-
Available to Order - This title is available to order, with delivery expected within 2 weeks
- Format:Paperback / softback
- Pages:104 pages, 25 Illustrations, color; 21 Illustrations, black and white; XII, 104 p. 46 illus., 25 ill
- Publisher:Springer Nature Switzerland AG
- Publication Date:15/02/2022
- Category:
- ISBN:9783030944810
Information
-
Available to Order - This title is available to order, with delivery expected within 2 weeks
- Format:Paperback / softback
- Pages:104 pages, 25 Illustrations, color; 21 Illustrations, black and white; XII, 104 p. 46 illus., 25 ill
- Publisher:Springer Nature Switzerland AG
- Publication Date:15/02/2022
- Category:
- ISBN:9783030944810