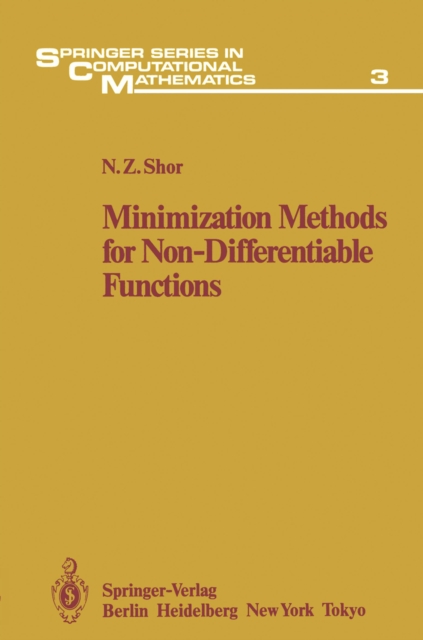
Minimization Methods for Non-Differentiable Functions PDF
by N.Z. Shor
Part of the Springer Series in Computational Mathematics series
Description
In recent years much attention has been given to the development of auto- matic systems of planning, design and control in various branches of the national economy.
Quality of decisions is an issue which has come to the forefront, increasing the significance of optimization algorithms in math- ematical software packages for al,ltomatic systems of various levels and pur- poses.
Methods for minimizing functions with discontinuous gradients are gaining in importance and the ~xperts in the computational methods of mathematical programming tend to agree that progress in the development of algorithms for minimizing nonsmooth functions is the key to the con- struction of efficient techniques for solving large scale problems.
This monograph summarizes to a certain extent fifteen years of the author's work on developing generalized gradient methods for nonsmooth minimization.
This work started in the department of economic cybernetics of the Institute of Cybernetics of the Ukrainian Academy of Sciences under the supervision of V.S.
Mikhalevich, a member of the Ukrainian Academy of Sciences, in connection with the need for solutions to important, practical problems of optimal planning and design.
In Chap. I we describe basic classes of nonsmooth functions that are dif- ferentiable almost everywhere, and analyze various ways of defining generalized gradient sets.
In Chap. 2 we study in detail various versions of the su bgradient method, show their relation to the methods of Fejer-type approximations and briefly present the fundamentals of e-subgradient methods.
Information
-
Download - Immediately Available
- Format:PDF
- Publisher:Springer Berlin Heidelberg
- Publication Date:06/12/2012
- Category:
- ISBN:9783642821189
Other Formats
- Paperback / softback from £57.89
Information
-
Download - Immediately Available
- Format:PDF
- Publisher:Springer Berlin Heidelberg
- Publication Date:06/12/2012
- Category:
- ISBN:9783642821189