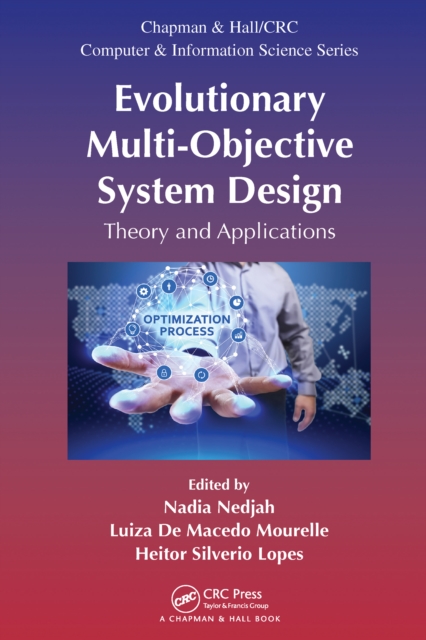
Evolutionary Multi-Objective System Design : Theory and Applications PDF
Edited by Nadia (State University of Rio de Janeiro, Brazil) Nedjah, Luiza (State Ubi of Rio de Janeiro, Brazil) De Macedo Mourelle, Heitor Silverio Lopes
Part of the Chapman & Hall/CRC Computer and Information Science Series series
Description
Real-world engineering problems often require concurrent optimization of several design objectives, which are conflicting in cases.
This type of optimization is generally called multi-objective or multi-criterion optimization.
The area of research that applies evolutionary methodologies to multi-objective optimization is of special and growing interest.
It brings a viable computational solution to many real-world problems.
Generally, multi-objective engineering problems do not have a straightforward optimal design.
These kinds of problems usually inspire several solutions of equal efficiency, which achieve different trade-offs.
Decision makers’ preferences are normally used to select the most adequate design.
Such preferences may be dictated before or after the optimization takes place.
They may also be introduced interactively at different levels of the optimization process.
Multi-objective optimization methods can be subdivided into classical and evolutionary.
The classical methods usually aim at a single solution while the evolutionary methods provide a whole set of so-called Pareto-optimal solutions.
Evolutionary Multi-Objective System Design: Theory and Applicationsprovides a representation of the state-of-the-art in evolutionary multi-objective optimization research area and related new trends.
It reports many innovative designs yielded by the application of such optimization methods.
It also presents the application of multi-objective optimization to the following problems: Embrittlement of stainless steel coated electrodes Learning fuzzy rules from imbalanced datasets Combining multi-objective evolutionary algorithms with collective intelligence Fuzzy gain scheduling control Smart placement of roadside units in vehicular networks Combining multi-objective evolutionary algorithms with quasi-simplex local search Design of robust substitution boxes Protein structure prediction problem Core assignment for efficient network-on-chip-based system design
Information
-
Download - Immediately Available
- Format:PDF
- Pages:242 pages, 63 Tables, black and white; 50 Illustrations, black and white
- Publisher:Taylor & Francis Inc
- Publication Date:15/07/2020
- Category:
- ISBN:9781498780292
Information
-
Download - Immediately Available
- Format:PDF
- Pages:242 pages, 63 Tables, black and white; 50 Illustrations, black and white
- Publisher:Taylor & Francis Inc
- Publication Date:15/07/2020
- Category:
- ISBN:9781498780292