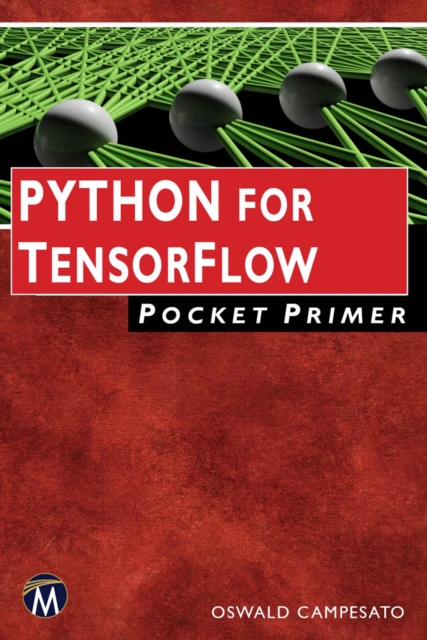
Description
As part of the best-selling PocketPrimer series, this book is designed to prepare programmers for machine learning and deep learning/TensorFlow topics.
It begins with a quick introduction to Python, followed by chapters that discuss NumPy, Pandas, Matplotlib, and scikit-learn.
The final two chapters contain an assortment of TensorFlow 1.x code samples, including detailed code samples for TensorFlowDataset (which is used heavily in TensorFlow 2 as well).
A TensorFlow Dataset refers to the classes in the tf.data.Dataset namespace that enables programmers to construct a pipeline of data by means of method chaining so-called lazy operators, e.g., map(), filter(), batch(), and so forth, based on data from one or more data sources. Companion files with source code are available for downloading from the publisher by writing info@merclearning.com. Features:A practical introduction to Python, NumPy, Pandas, Matplotlib, and introductory aspects of TensorFlow 1.xContains relevant NumPy/Pandas code samples that are typical in machine learning topics, and also useful TensorFlow 1.x code samples for deep learning/TensorFlow topicsIncludes many examples of TensorFlow Dataset APIs with lazy operators, e.g., map(), filter(), batch(), take() and also method chaining such operatorsAssumes the reader has very limited experienceCompanion files with all of the source code examples (download from the publisher)
Information
-
Download - Immediately Available
- Format:PDF
- Pages:218 pages
- Publisher:Mercury Learning & Information
- Publication Date:23/05/2019
- Category:
- ISBN:9781683923633
Other Formats
- Paperback / softback from £29.95
Information
-
Download - Immediately Available
- Format:PDF
- Pages:218 pages
- Publisher:Mercury Learning & Information
- Publication Date:23/05/2019
- Category:
- ISBN:9781683923633