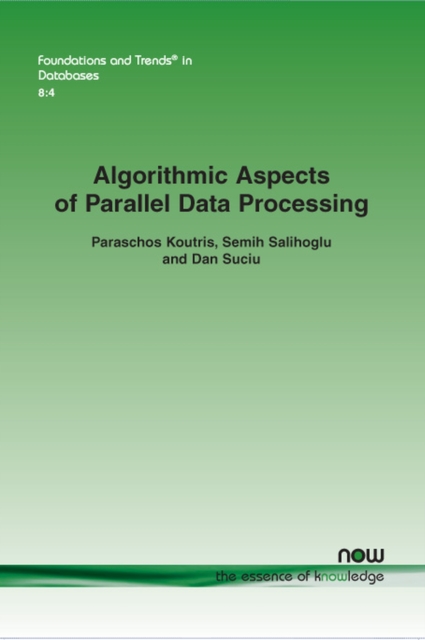
Algorithmic Aspects of Parallel Data Processing Paperback / softback
by PARASCHOS KOUTRIS, Semih Salihoglu, Dan Suciu
Part of the Foundations and Trends in Databases series
Paperback / softback
Description
The last decade has seen a huge and growing interest in processing large data sets on large distributed clusters.
This trend began with the MapReduce framework, and has been widely adopted by several other systems, including PigLatin, Hive, Scope, Dremmel, Spark and Myria to name a few.
While the applications of such systems are diverse (for example, machine learning, data analytics), most involve relatively standard data processing tasks like identifying relevant data, cleaning, filtering, joining, grouping, transforming, extracting features, and evaluating results.
This has generated great interest in the study of algorithms for data processing on large distributed clusters.
Algorithmic Aspects of Parallel Data Processing discusses recent algorithmic developments for distributed data processing.
It uses a theoretical model of parallel processing called the Massively Parallel Computation (MPC) model, which is a simplification of the BSP model where the only cost is given by the amount of communication and the number of communication rounds.
The survey studies several algorithms for multi-join queries, sorting, and matrix multiplication.
It discusses their relationships and common techniques applied across the different data processing tasks.
Information
-
Available to Order - This title is available to order, with delivery expected within 2 weeks
- Format:Paperback / softback
- Pages:144 pages
- Publisher:now publishers Inc
- Publication Date:22/02/2018
- Category:
- ISBN:9781680834062
Information
-
Available to Order - This title is available to order, with delivery expected within 2 weeks
- Format:Paperback / softback
- Pages:144 pages
- Publisher:now publishers Inc
- Publication Date:22/02/2018
- Category:
- ISBN:9781680834062