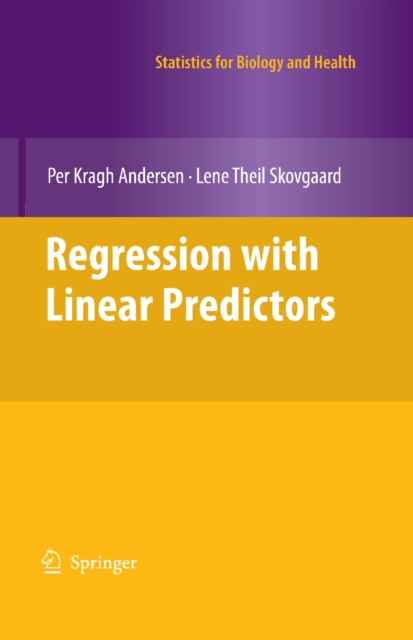
Regression with Linear Predictors PDF
by Per Kragh Andersen, Lene Theil Skovgaard
Part of the Statistics for Biology and Health series
Description
This is a book about regression analysis, that is, the situation in statistics where the distribution of a response (or outcome) variable is related to - planatory variables (or covariates).
This is an extremely common situation in the application of statistical methods in many ?elds, andlinear regression,- gistic regression, and Cox proportional hazards regression are frequently used for quantitative, binary, and survival time outcome variables, respectively.
Several books on these topics have appeared and for that reason one may well ask why we embark on writing still another book on regression.
We have two main reasons for doing this: 1. First, we want to highlightsimilaritiesamonglinear,logistic,proportional hazards,andotherregressionmodelsthatincludealinearpredictor.
These modelsareoftentreatedentirelyseparatelyintextsinspiteofthefactthat alloperationsonthemodelsdealingwiththelinearpredictorareprecisely the same, including handling of categorical and quantitative covariates, testing for linearity and studying interactions. 2. Second, we want to emphasize that, for any type of outcome variable, multiple regression models are composed of simple building blocks that areaddedtogetherinthelinearpredictor:thatis,t-tests,one-wayanalyses of variance and simple linear regressions for quantitative outcomes, 2×2, 2×(k+1) tables and simple logistic regressions for binary outcomes, and 2-and (k+1)-sample logrank testsand simple Cox regressionsfor survival data.
Thishastwoconsequences. Allthesesimpleandwellknownmethods can be considered as special cases of the regression models.
On the other hand, the e?ect of a single explanatory variable in a multiple regression model can be interpreted in a way similar to that obtained in the simple analysis, however, now valid only forthe other explanatory variables in the model "held ?xed".
Information
-
Download - Immediately Available
- Format:PDF
- Publisher:Springer New York
- Publication Date:15/07/2010
- Category:
- ISBN:9781441971708
Information
-
Download - Immediately Available
- Format:PDF
- Publisher:Springer New York
- Publication Date:15/07/2010
- Category:
- ISBN:9781441971708