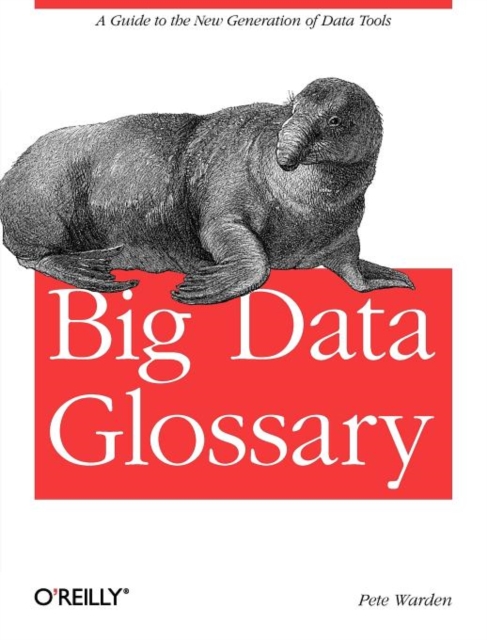
Big Data Glossary Paperback / softback
by Pete Warden
Paperback / softback
Description
There's been a massive amount of innovation in data tools over the last few years, thanks to a few key trends: * *Learning from the web*.
Techniques originally developed by website developers coping with scaling issues are increasingly being applied to other domains. * *CS+?=$$$*. Google have proven that research techniques from computer science can be effective at solving problems and creating value in many real-world situations.
That's led to increased interest in cross-pollination and investment in academic research from commercial organizations. * *Cheap hardware*. Now that machines with a decent amount of processing power can be hired for just a few cents an hour, many more people can afford to do large-scale data processing.
They can't afford the traditional high prices of professional data software though, so they've turned to open-source alternatives.
These trends have led to a Cambrian Explosion of new tools, which means when you're planning a new data project you have a lot to choose from.
This guide aims to help you make those choices by describing each tool from the perspective of a developer looking to use them in an application. Wherever possible, this will be from my first-hand experiences, or from colleagues who have used the systems in production environments.
I've made a deliberate choice to include my own opinions and impressions, so you should see this guide as a starting point for exploring the tools, not the final word.
I'll do my best to explain what I like about each service but your tastes and requirements may well be quite different.
Since the goal is to help experienced engineers navigate the new data landscape, the guide only covers tools that have been created or risen to prominence in the last few years.
For example, PostGres is not covered because it's been widely used for over a decade, but its Greenplum derivative is newer and less well-known, so it is included.
Information
-
Available to Order - This title is available to order, with delivery expected within 2 weeks
- Format:Paperback / softback
- Pages:60 pages, Illustrations, map
- Publisher:O'Reilly Media
- Publication Date:25/10/2011
- Category:
- ISBN:9781449314590
Other Formats
- EPUB from £9.59
- PDF from £8.40
Information
-
Available to Order - This title is available to order, with delivery expected within 2 weeks
- Format:Paperback / softback
- Pages:60 pages, Illustrations, map
- Publisher:O'Reilly Media
- Publication Date:25/10/2011
- Category:
- ISBN:9781449314590