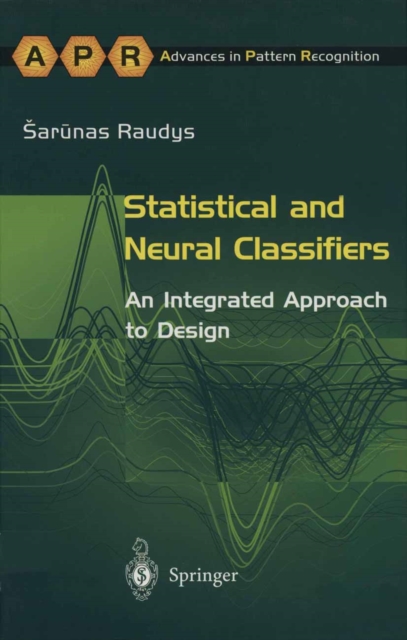
Statistical and Neural Classifiers : An Integrated Approach to Design PDF
by Sarunas Raudys
Part of the Advances in Computer Vision and Pattern Recognition series
Description
Automatic (machine) recognition, description, classification, and groupings of patterns are important problems in a variety of engineering and scientific disciplines such as biology, psychology, medicine, marketing, computer vision, artificial intelligence, and remote sensing.
Given a pattern, its recognition/classification may consist of one of the following two tasks: (1) supervised classification (also called discriminant analysis); the input pattern is assigned to one of several predefined classes, (2) unsupervised classification (also called clustering); no pattern classes are defined a priori and patterns are grouped into clusters based on their similarity.
Interest in the area of pattern recognition has been renewed recently due to emerging applications which are not only challenging but also computationally more demanding (e. g. , bioinformatics, data mining, document classification, and multimedia database retrieval).
Among the various frameworks in which pattern recognition has been traditionally formulated, the statistical approach has been most intensively studied and used in practice.
More recently, neural network techniques and methods imported from statistical learning theory have received increased attention.
Neural networks and statistical pattern recognition are two closely related disciplines which share several common research issues.
Neural networks have not only provided a variety of novel or supplementary approaches for pattern recognition tasks, but have also offered architectures on which many well-known statistical pattern recognition algorithms can be mapped for efficient (hardware) implementation.
On the other hand, neural networks can derive benefit from some well-known results in statistical pattern recognition.
Information
-
Download - Immediately Available
- Format:PDF
- Publisher:Springer London
- Publication Date:06/12/2012
- Category:
- ISBN:9781447103592
Other Formats
- Paperback / softback from £104.05
- Hardback from £120.95
Information
-
Download - Immediately Available
- Format:PDF
- Publisher:Springer London
- Publication Date:06/12/2012
- Category:
- ISBN:9781447103592