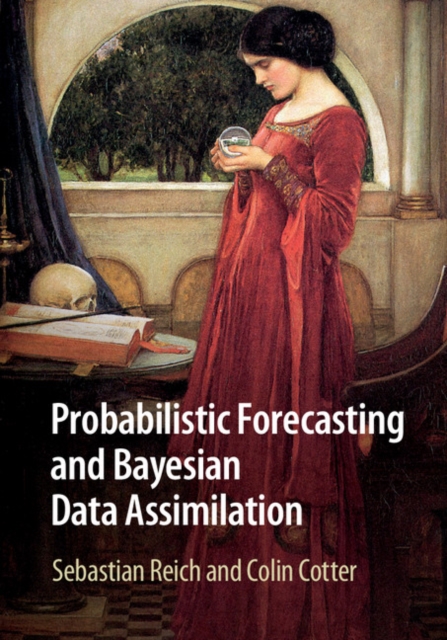
Probabilistic Forecasting and Bayesian Data Assimilation PDF
by Sebastian Reich, Colin Cotter
Description
In this book the authors describe the principles and methods behind probabilistic forecasting and Bayesian data assimilation.
Instead of focusing on particular application areas, the authors adopt a general dynamical systems approach, with a profusion of low-dimensional, discrete-time numerical examples designed to build intuition about the subject.
Part I explains the mathematical framework of ensemble-based probabilistic forecasting and uncertainty quantification.
Part II is devoted to Bayesian filtering algorithms, from classical data assimilation algorithms such as the Kalman filter, variational techniques, and sequential Monte Carlo methods, through to more recent developments such as the ensemble Kalman filter and ensemble transform filters.
The McKean approach to sequential filtering in combination with coupling of measures serves as a unifying mathematical framework throughout Part II.
Assuming only some basic familiarity with probability, this book is an ideal introduction for graduate students in applied mathematics, computer science, engineering, geoscience and other emerging application areas.
Information
-
Download - Immediately Available
- Format:PDF
- Publisher:Cambridge University Press
- Publication Date:14/05/2015
- Category:
- ISBN:9781316309490
Other Formats
- EPUB from £36.54
Information
-
Download - Immediately Available
- Format:PDF
- Publisher:Cambridge University Press
- Publication Date:14/05/2015
- Category:
- ISBN:9781316309490