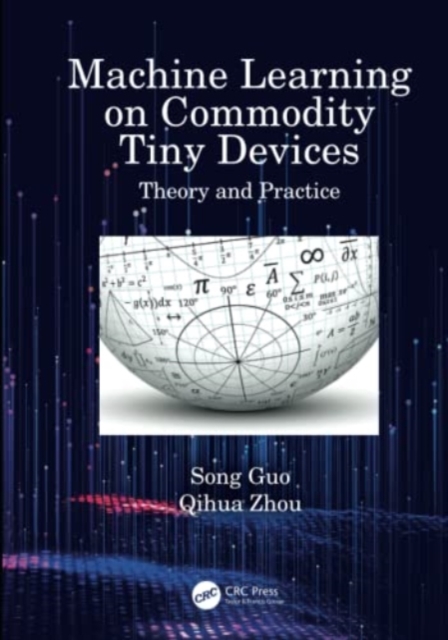
Machine Learning on Commodity Tiny Devices : Theory and Practice Hardback
by Song Guo, Qihua Zhou
Hardback
Description
This book aims at the tiny machine learning (TinyML) software and hardware synergy for edge intelligence applications.
This book presents on-device learning techniques covering model-level neural network design, algorithm-level training optimization and hardware-level instruction acceleration. Analyzing the limitations of conventional in-cloud computing would reveal that on-device learning is a promising research direction to meet the requirements of edge intelligence applications.
As to the cutting-edge research of TinyML, implementing a high-efficiency learning framework and enabling system-level acceleration is one of the most fundamental issues.
This book presents a comprehensive discussion of the latest research progress and provides system-level insights on designing TinyML frameworks, including neural network design, training algorithm optimization and domain-specific hardware acceleration.
It identifies the main challenges when deploying TinyML tasks in the real world and guides the researchers to deploy a reliable learning system. This book will be of interest to students and scholars in the field of edge intelligence, especially to those with sufficient professional Edge AI skills.
It will also be an excellent guide for researchers to implement high-performance TinyML systems.
Information
-
Only a few left - usually despatched within 24 hours
- Format:Hardback
- Pages:250 pages, 7 Tables, black and white; 36 Line drawings, black and white; 20 Halftones, black and whi
- Publisher:Taylor & Francis Ltd
- Publication Date:13/12/2022
- Category:
- ISBN:9781032374239
Other Formats
- PDF from £62.99
- EPUB from £62.99
Information
-
Only a few left - usually despatched within 24 hours
- Format:Hardback
- Pages:250 pages, 7 Tables, black and white; 36 Line drawings, black and white; 20 Halftones, black and whi
- Publisher:Taylor & Francis Ltd
- Publication Date:13/12/2022
- Category:
- ISBN:9781032374239